Cities are becoming increasingly data-driven. Advanced Driver-Assistance Systems (ADAS) and the Internet of Things (IoT) are generating a wealth of data that can be used to improve urban planning.
ADAS has transformed driving from a solely human activity into a human-machine collaboration. Powered by AI and machine learning, these systems are learning and evolving and increasingly driving us toward a future of autonomous vehicles.
Coupled with the interconnectedness of the IoT, ADAS is generating a goldmine of data that can be used to paint a high-resolution picture of our cities’ mobility patterns. This data can be used to engineer more efficient transport networks, combat climate change, and enhance pedestrian safety.
Machine learning is another key technology that is transforming city planning. Machine learning can be used to evolve infrastructure, optimize traffic management, and even predict future patterns. This allows cities to be proactive rather than reactive.
However, there are challenges to using data and machine learning for city planning. Cities are complex entities, and their data is high-dimensional and time-dependent. It requires advanced data processing capabilities to analyze and translate into actionable insights.
Privacy and security are also concerns. As we collect and analyze more data, it is important to ensure that citizens’ privacy is respected and that data is secure.
Finally, the gap between innovation and legislation must be bridged. Regulations must evolve in line with technological advancement to enable the full exploitation of the ADAS and IoT potential without compromising on safety.
The smart cities in action
Consider the case of Boston, a city that has fully embraced the potential of ADAS and IoT. As per a report from the Boston Smart City Lab (2023), since the implementation of data-driven traffic management systems that leverage ADAS and IoT, the city has seen a 20% reduction in peak-hour congestion. Furthermore, by using data from these systems to identify and rectify accident-prone locations, traffic-related accidents have been reduced by 15%.
On the environmental front, San Francisco’s ‘Smart City Initiative’ is a beacon of how IoT and ADAS can help mitigate climate change. Utilizing data from these technologies, the city has optimized traffic flow and public transportation routes, resulting in a 25% decrease in CO2 emissions from the transportation sector over the past five years, according to the Environmental Monitoring Bureau of San Francisco (2023).
Internationally, Copenhagen has taken the lead in integrating IoT technology within its urban planning. As per the Copenhagen Connectivity Report (2023), the city has deployed a network of sensors that interact with ADAS-equipped vehicles. This network guides traffic, reducing congestion and decreasing commute times by 30%, enhancing the overall quality of life for the city’s residents.
The promise of smart cities
Despite the challenges, the promise of smart cities is clear. Data analytics and machine learning are not just technological trends but crucial tools for smart city planning. With continued collaboration and innovation, we can transform our cities into sustainability, safety, and efficiency hubs.
To ensure a future where cities work for everyone, we must be ready to learn from the data around us, adapt our approaches, and revolutionize our cityscapes. Together, we can drive this change.
Here are some of the benefits of using data and machine learning for city planning:
- More efficient transport networks: Data can be used to identify traffic hotspots and optimize traffic flow. This can lead to shorter commute times and reduced emissions.
- Improved pedestrian safety: Data can be used to identify areas where pedestrians are at risk and implement safety measures. This can lead to a reduction in pedestrian injuries and fatalities.
- Enhanced sustainability: Data can be used to identify ways to reduce energy consumption and waste. This can lead to a more sustainable city.
- Improved quality of life: Data can be used to identify ways to improve the quality of life for residents. This can include improving air quality, reducing crime, and providing more affordable housing.
But as great as these technologies are for smart city planning, they come with a few challenges, such as:
Data privacy: As we collect and analyze more data, it is important to respect citizens’ privacy.
Data security: Data is a valuable asset, and protecting it from unauthorized access is important.
Lack of expertise: Not all cities have the expertise or resources to use data and machine learning effectively.
Cost: Data and machine learning can be expensive to implement and maintain.
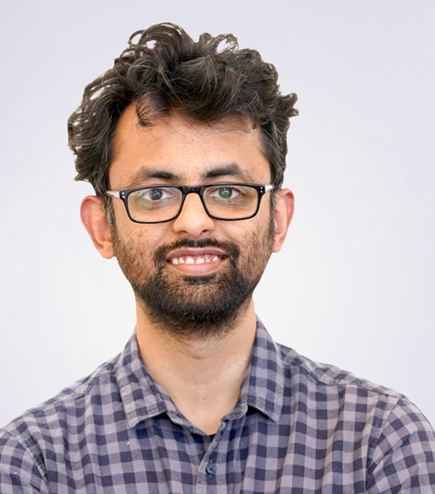
About the author:
Gautam BT is the CEO and Co-Founder of Bytebeam, a technology company driving innovation and digital transformation. With his visionary leadership, Gautam has spearheaded Bytebeam’s growth and positioned it as a key player in the industry. His expertise lies in leveraging emerging technologies to create impactful solutions for businesses and organisations.